Skip
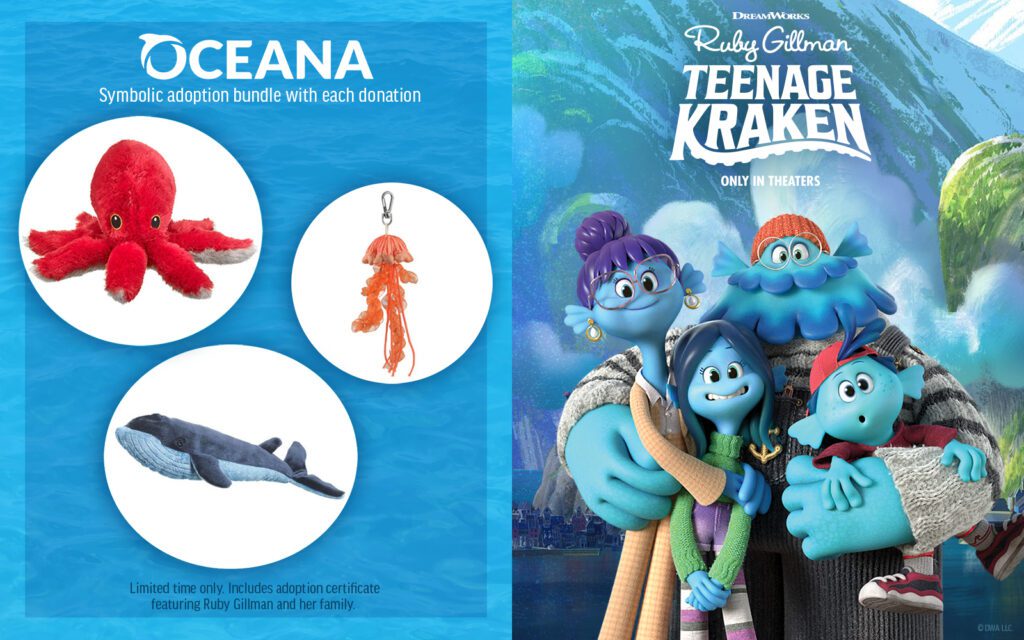
The rapid evolution of technology has dramatically altered the landscape of various industries, and one of the most significant transformations has been in the field of data analysis and interpretation. With the advent of advanced computational capabilities and sophisticated algorithms, the ability to parse through vast amounts of data to derive meaningful insights has become more efficient and accessible. This shift has profound implications for businesses, research institutions, and individuals seeking to make informed decisions based on data-driven strategies.
At the heart of this revolution is the concept of machine learning, a subset of artificial intelligence that enables systems to learn from data, identify patterns, and make decisions with minimal human intervention. Machine learning algorithms can be broadly categorized into supervised, unsupervised, and reinforcement learning, each with its unique applications and advantages. Supervised learning, for instance, involves training algorithms on labeled data to predict outcomes for new, unseen data. This approach has been instrumental in image classification, speech recognition, and predictive analytics. Unsupervised learning, on the other hand, focuses on discovering hidden patterns in unlabeled data, making it particularly useful for clustering and dimensionality reduction. Reinforcement learning takes a different approach, where algorithms learn to take actions in an environment to maximize a reward, finding applications in robotics and game theory.
One of the most compelling advantages of machine learning is its ability to handle complex, high-dimensional data that would be impractical or impossible for humans to analyze manually. This capability has opened up new avenues for research and innovation, particularly in fields like healthcare, finance, and climate science, where the volume and complexity of data are staggering. For example, in healthcare, machine learning can be used to analyze medical images, patient histories, and genetic data to diagnose diseases more accurately and earlier than traditional methods. Similarly, in finance, machine learning algorithms can analyze market trends, news, and social media sentiment to predict stock prices and portfolio performance.
Despite the numerous benefits and advancements brought about by machine learning, there are also challenges and ethical considerations that need to be addressed. One of the significant concerns is the issue of bias in machine learning models. If the training data reflects existing societal biases, the models will learn and perpetuate these biases, leading to unfair outcomes in applications such as hiring, lending, and law enforcement. Furthermore, the interpretability of machine learning models is another area of concern. As models become more complex, understanding why a particular decision was made becomes increasingly difficult, which can hinder trust and accountability in critical applications.
To mitigate these challenges, researchers and practitioners are focusing on developing more transparent and explainable machine learning models. Techniques such as feature attribution and model interpretability methods are being explored to provide insights into the decision-making processes of complex algorithms. Additionally, there is a growing emphasis on ensuring that training data is diverse and representative, and on developing algorithms that can detect and mitigate bias.
The future of machine learning holds much promise, with ongoing research aimed at pushing the boundaries of what is possible. The integration of machine learning with other technologies, such as the Internet of Things (IoT), cloud computing, and quantum computing, is expected to unlock new capabilities and applications. For instance, the combination of machine learning with IoT can enable smart cities and homes, where devices and infrastructure can adapt and respond based on real-time data analysis. Similarly, the advent of quantum machine learning could lead to breakthroughs in fields like cryptography, optimization problems, and drug discovery.
In conclusion, machine learning has emerged as a powerful tool for extracting insights from data, with far-reaching implications across various sectors. As this field continues to evolve, addressing the challenges of bias, interpretability, and ethical use will be crucial to ensuring that the benefits of machine learning are realized for the betterment of society as a whole.
Practical Applications of Machine Learning
Machine learning has numerous practical applications across different industries. Here are a few examples:
- Healthcare: Machine learning can be used for disease diagnosis, personalized medicine, and drug discovery.
- Finance: It can analyze financial data to predict stock prices, detect fraudulent transactions, and manage risk.
- Customer Service: Chatbots and virtual assistants use machine learning to provide personalized support to customers.
- Autonomous Vehicles: Machine learning algorithms enable self-driving cars to interpret sensory data and make decisions in real-time.
Technical Breakdown of Machine Learning Algorithms
Understanding the technical aspects of machine learning algorithms is crucial for their effective implementation. This involves delving into the mathematical foundations, computational complexity, and optimization techniques used in these algorithms. For instance, gradient descent is a fundamental optimization algorithm used in many machine learning models to minimize the loss function.
Future Trends in Machine Learning
As machine learning continues to advance, several trends are expected to shape its future. These include the increased use of reinforcement learning, the development of more sophisticated explainable AI techniques, and the integration of machine learning with edge computing to reduce latency and improve real-time decision-making.
Decision Framework for Implementing Machine Learning
When considering the implementation of machine learning in an organization, several factors need to be evaluated, including the availability of data, the complexity of the problem to be solved, the computational resources required, and the potential return on investment. A structured decision framework can help guide this process, ensuring that the adoption of machine learning aligns with business objectives and strategy.
Resource Guide for Machine Learning
For those looking to dive deeper into machine learning, there are numerous resources available, including online courses, textbooks, and research papers. Some of the most popular resources include Andrew Ng’s Machine Learning course on Coursera, the book “Pattern Recognition and Machine Learning” by Christopher Bishop, and research journals like the Journal of Machine Learning Research.
Common Misconceptions About Machine Learning
Despite its growing popularity, there are several misconceptions about machine learning. One common myth is that machine learning requires a significant amount of labeled data, which is not always the case, especially with unsupervised and semi-supervised learning techniques. Another myth is that machine learning models are always more accurate than traditional statistical models, which depends on the specific problem and data.
What is the primary difference between supervised and unsupervised machine learning?
+Supervised learning involves training algorithms on labeled data to predict outcomes for new data, whereas unsupervised learning focuses on discovering patterns in unlabeled data without prior knowledge of the expected outcome.
How can bias in machine learning models be mitigated?
+Bias in machine learning models can be mitigated by ensuring that the training data is diverse and representative, using techniques to detect and correct for bias, and implementing algorithms that are designed to reduce bias.
What are some of the future trends expected in machine learning?
+Future trends in machine learning include the increased use of reinforcement learning, the development of more sophisticated explainable AI techniques, and the integration of machine learning with edge computing.
As we continue to navigate the complexities and opportunities presented by machine learning, it’s essential to approach this technology with a balanced perspective, recognizing both its potential to drive innovation and its need for responsible development and use. By doing so, we can harness the power of machine learning to create a future that is more informed, more efficient, and more equitable for all.