Maria Zhang Deepfake Detection
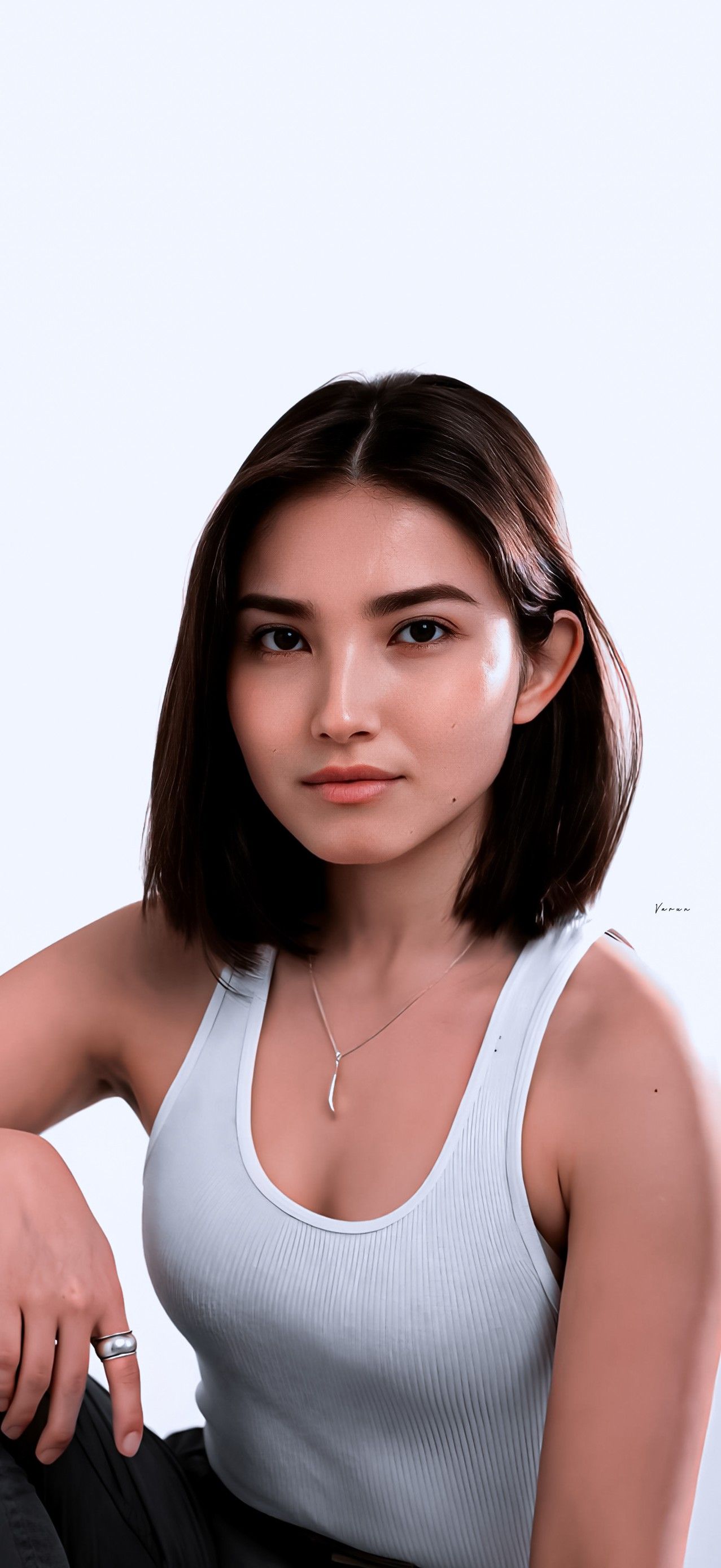
The advent of deepfake technology has raised significant concerns about the potential for malicious use of fake audio and video recordings. One of the key figures at the forefront of deepfake detection is Maria Zhang, a renowned expert in the field of artificial intelligence and machine learning. Zhang’s work has focused on developing innovative methods for identifying and mitigating the effects of deepfakes, and her research has garnered considerable attention from academia, industry, and government agencies.
At the heart of Zhang’s approach to deepfake detection is a deep understanding of the underlying technology used to create these fake recordings. Deepfakes rely on advanced machine learning algorithms, such as generative adversarial networks (GANs) and variational autoencoders (VAEs), to generate highly realistic audio and video simulations. However, these algorithms also leave behind subtle artifacts and inconsistencies that can be exploited for detection purposes. Zhang’s work has centered on identifying and analyzing these artifacts, developing sophisticated methods for distinguishing between genuine and fake recordings.
One of the primary challenges in deepfake detection is the rapid evolution of the technology itself. As deepfake creation tools become increasingly sophisticated, the task of detecting them becomes more complex. Zhang has addressed this challenge by developing adaptive detection methods that can learn from emerging threats and improve their accuracy over time. Her approach involves training machine learning models on large datasets of genuine and fake recordings, allowing the models to learn the subtle patterns and anomalies that distinguish between the two.
A key aspect of Zhang’s research is the emphasis on multimodal analysis. Deepfakes often involve both audio and video components, and analyzing these components in isolation can be insufficient for effective detection. Zhang’s methods integrate both audio and video analysis, examining the interactions and correlations between the two modalities to identify inconsistencies and anomalies. This multimodal approach has been shown to significantly improve detection accuracy, particularly in cases where the fake recording is highly realistic.
In addition to her technical contributions, Zhang has also been a vocal advocate for the importance of deepfake detection in maintaining the integrity of digital media. She has emphasized the potential consequences of unchecked deepfake dissemination, including the erosion of trust in digital media, the spread of misinformation, and the potential for malicious actors to exploit deepfakes for financial or political gain. Zhang’s work has highlighted the need for a concerted effort to develop and deploy effective deepfake detection tools, and her research has played a significant role in shaping the ongoing conversation around this critical issue.
According to Zhang, "The detection of deepfakes is a constantly evolving challenge, as the technology used to create them continues to advance. However, by developing sophisticated methods for analyzing the artifacts and inconsistencies left behind by these algorithms, we can improve our ability to distinguish between genuine and fake recordings."
Zhang’s research has also explored the potential applications of deepfake detection in various domains, including cybersecurity, law enforcement, and social media moderation. Her work has shown that effective deepfake detection can play a critical role in preventing the spread of misinformation, protecting individuals from identity theft and fraud, and maintaining the integrity of digital evidence.
Steps for Deepfake Detection:
- Data Collection: Gather a large dataset of genuine and fake recordings, including audio and video components.
- Machine Learning Model Training: Train machine learning models on the collected dataset to learn the patterns and anomalies that distinguish between genuine and fake recordings.
- Multimodal Analysis: Integrate both audio and video analysis to examine the interactions and correlations between the two modalities.
- Anomaly Detection: Apply anomaly detection techniques to identify inconsistencies and anomalies in the analyzed recordings.
- Verification and Validation: Verify and validate the detection results using human evaluators and additional analysis tools.
As the landscape of deepfake technology continues to evolve, Zhang’s work remains at the forefront of the effort to develop effective detection methods. Her research has demonstrated the potential for machine learning and multimodal analysis to improve deepfake detection accuracy, and her advocacy has highlighted the critical importance of addressing this issue. As we move forward in this rapidly changing environment, Zhang’s contributions will undoubtedly play a significant role in shaping the future of deepfake detection and mitigation.
Pros and Cons of Deepfake Detection Methods:
Method | Pros | Cons |
---|---|---|
Machine Learning | High accuracy, adaptability to emerging threats | Requires large datasets, can be computationally intensive |
Multimodal Analysis | Improves detection accuracy, examines interactions between modalities | Can be complex to implement, requires expertise in multiple domains |
Anomaly Detection | Effective in identifying inconsistencies and anomalies | Can generate false positives, requires careful tuning of parameters |
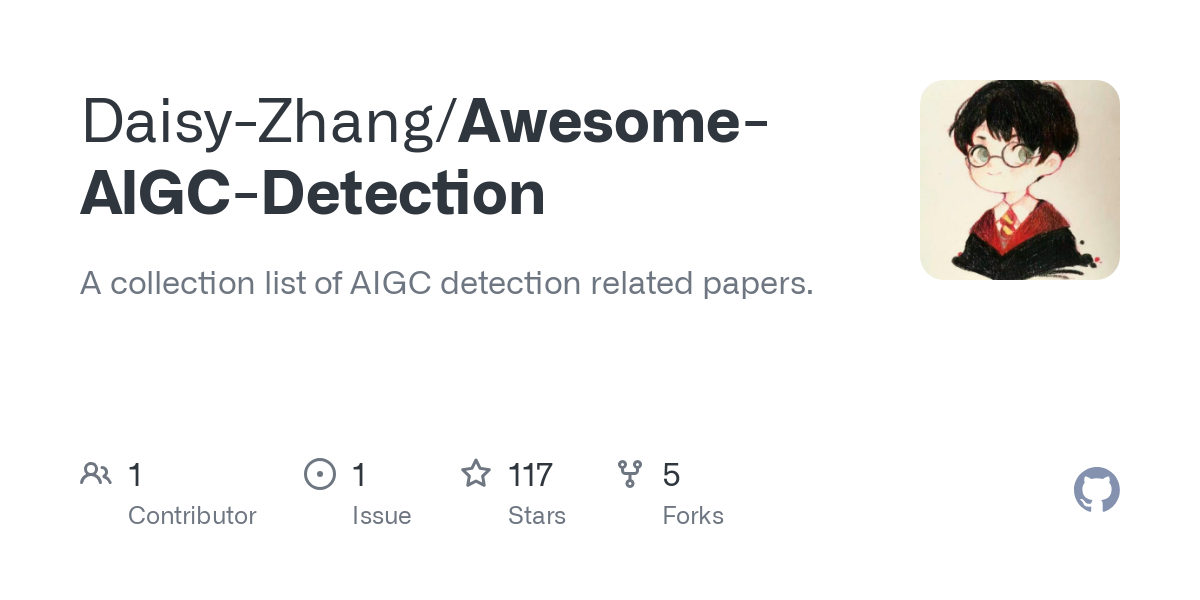
In conclusion, Maria Zhang’s work on deepfake detection has made significant contributions to the field of artificial intelligence and machine learning. Her research has demonstrated the potential for effective deepfake detection using machine learning and multimodal analysis, and her advocacy has highlighted the importance of addressing this critical issue. As we continue to navigate the complex landscape of deepfake technology, Zhang’s work will undoubtedly play a critical role in shaping the future of deepfake detection and mitigation.
What is deepfake detection, and why is it important?
+Deepfake detection refers to the process of identifying and distinguishing between genuine and fake audio and video recordings. It is important because deepfakes can be used to spread misinformation, commit identity theft, and compromise the integrity of digital media.
What are the challenges in detecting deepfakes, and how can they be addressed?
+The challenges in detecting deepfakes include the rapid evolution of the technology, the complexity of the algorithms used, and the potential for deepfakes to be highly realistic. These challenges can be addressed by developing adaptive detection methods, integrating multimodal analysis, and improving the accuracy of machine learning models.
What role can machine learning play in deepfake detection, and what are its limitations?
+Machine learning can play a significant role in deepfake detection by improving the accuracy of detection models and adapting to emerging threats. However, its limitations include the requirement for large datasets, the potential for overfitting, and the need for careful tuning of parameters.